To view the full content, login to your account,
or start your 30-day FREE Trial today.
FREE TRIAL
LOGIN
Forgot Password?
Explore some of our unlocked OE Originals below!
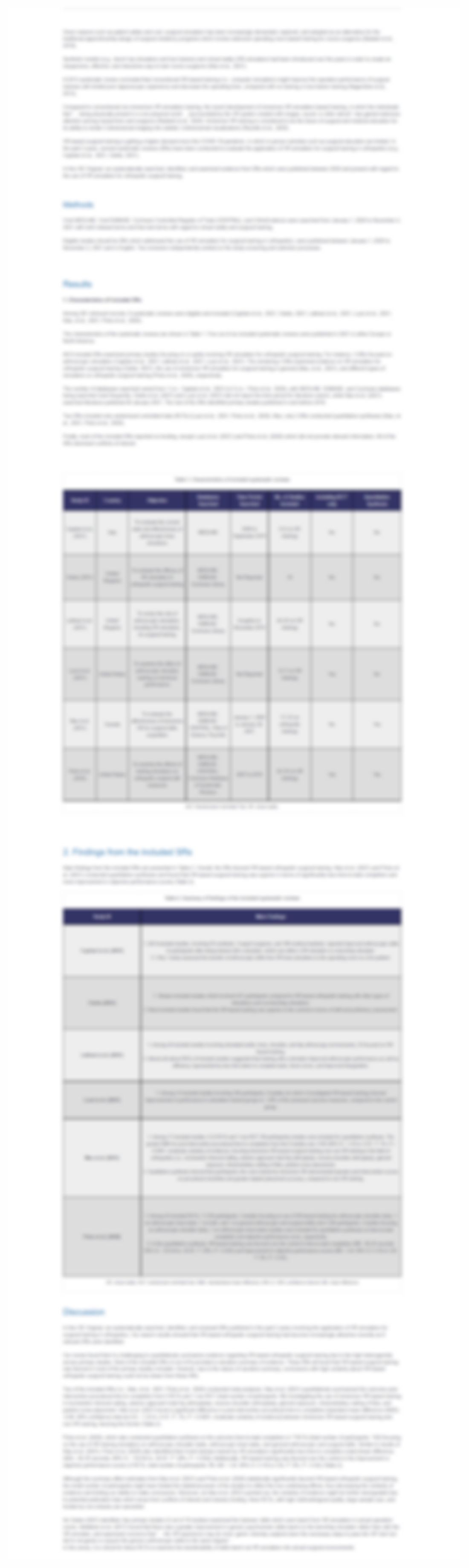
Join the Conversation
Please Login or Join to leave comments.
Physician - United States
Machine Learning presents significant potential to change the learning curve for surgeons, first in their decision-making and second in their actual surgery. The problem is that teaching a machine an approach is a bit like teaching religion. There may be many solutions to a given problem and some are based in evidence and some in eminence. That said, it is a worthy cause to work toward safety in surgery the same as has been done by the aviation industry as well. This is an area of great interest for me. Thank you for this article.