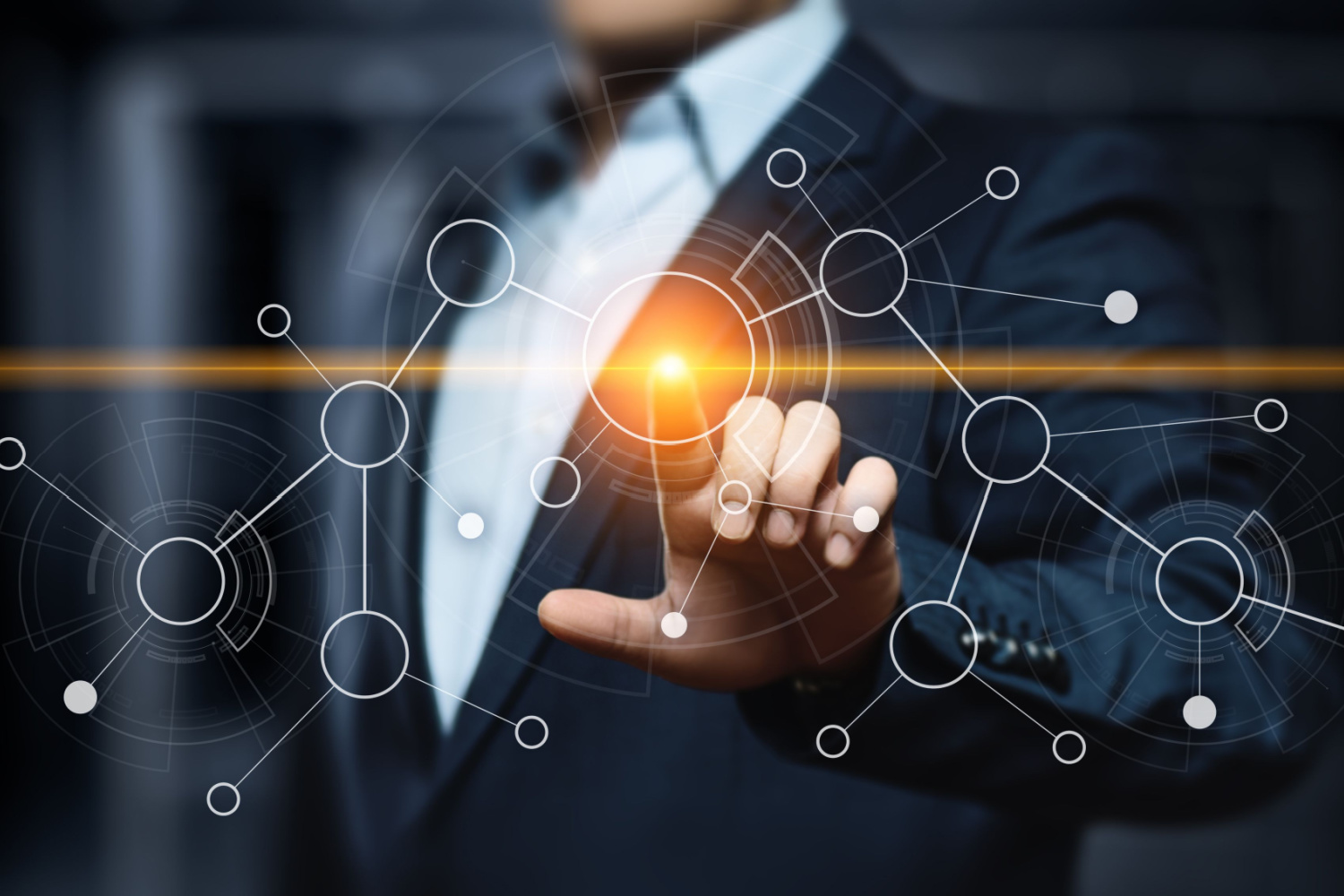
Beyond Impact Factor: Introducing OE High Impact - a Machine Learning Powered Metric of Article Influence
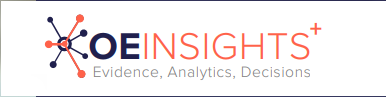
Beyond Impact Factor: Introducing OE High Impact - a Machine Learning Powered Metric of Article Influence
Vol: | Issue: | Number: | ISSN#: 2563-5972
September 2, 2022 | Article No. 105
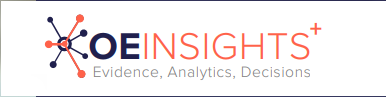
Beyond Impact Factor: Introducing OE High Impact - a Machine Learning Powered Metric of Article Influence
September 2, 2022 | Article No. 140
Mohit Bhandari MD, PhD
Joseph Silburt PhD
Insights
- OrthoEvidence presents OE High Impact, an upcoming feature to help readers identify impactful work at the time of their publication.
- We developed a neural network capable of predicting a paper’s future article citations based both on what the article says, and where it was published.
- Model predictions correlate 1.83-fold more with an article’s real-world citations than journal impact factor alone.
- The model is seemingly more sensitive to an article’s content, then it is to where it was published, in predicting an article’s citations.

"What matters absolutely is the scientific content of a paper, and nothing will substitute for either knowing or reading it."
Dr. Sydney Brenner, 1995
The Problem
As the volume of medical research continues to grow at an exponential rate, clinicians and researchers face an important yet often intractable problem, how do we stay up-to-date on important findings?
One common approach, born in the pre-internet age, is focusing largely on impact factor(1). Given time limitations, it might seem prudent to focus attention almost exclusively on esteemed or high-impact journals. However, a journal’s impact factor is largely driven by the success of their top performing articles, and thus, often it does not truly reflect the performance of an “average” article (2). More to the point, there is no doubt that many of the most influential works in orthopedics are found outside the New England Journal of Medicine (3). Ultimately it is not where a study is published, but what a study reveals that defines its impact (2).
Moving beyond impact factor, OE has aimed to leverage advances in machine learning to better predict an article’s potential impact at the time of publication. We present OE High Impact, a machine learning powered metric that serves as a better representation of an article’s potential importance than journal impact factor alone. Much of this improvement can be derived from the fact that OE High Impact makes predictions based on the content in the article itself.
The Model
In developing our neural network, we first generated a dataset of over 20,000 orthopedic clinical research articles from publicly available data sources. As an important caveat, journal metrics and article citations present in this dataset are slightly different from the “official” metrics calculated by the Journal of Citation Reports.
We subsequently trained a neural network to predict how many citations an article would receive based on both the article’s textual content (e.g., the article title, abstract text), and metrics on the article’s publishing journal (e.g., average citations per year, published articles per year, etc.). At the heart of this process is an inhouse developed “language model” which can convert the textual content present in an article into a numerical representation. In our final out-of-sample test-set, the citation prediction model was able to achieve a 1.72-fold stronger correlation with an article’s real-world citations than journal citation metrics alone (e.g., average citations per year) (Exhibit 1).
.png)
Exhibit 1: The OE citation prediction model shows a stronger correlation between predicted and actual number of article citations than impact factor alone.
Using the OE citation prediction model, we identified a cut-off, to discern articles which are likely to be highly cited for those that are not. We called this threshold OE High Impact. OE High Impact boasts a high precision – 72% of OE High Impact articles lie within the top 75th percent of cited articles, and 90% OE High Impact articles lie within the top 50th percentile of cited articles (Exhibit 2A). Thus, readers can be confident that OE High Impact articles are meritorious and worth reading. Notably, these articles come from a range of journals spanning the impact factor spectrum (Exhibit 2B). The median average citations per article of OE High Impact publishing journals is 3.89, illustrating that impactful research is not restricted to the pages of high-impact journals.
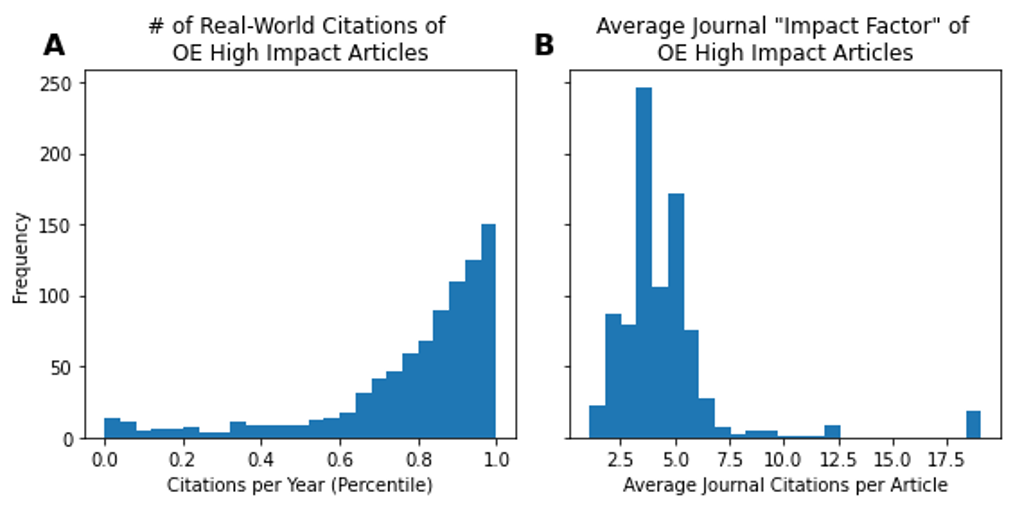
Exhibit 2: Articles identified as having an OE High Impact (A) are amongst the most cited articles, (B) but are published in a wide range of journals that are not reserved to “high impact journals”.
What are OE High Impact’s decisions based on?
Instead of being purely driven by the publishing journal, the OE citation prediction model is strongly dependent on an article’s content. As a “black box”, the neural network that powers OE High Impact cannot be simply asked to report a relative risk or odds ratio to confirm the relative importance of an article’s content, or a journal’s impact factor. However, we can still garner clues as to how it makes decisions by perturbing it. We therefore conducted two sensitivity analyses. In Exhibit 3A, we mutated every article such that it was published in a high-impact journal, median impact journal, and low-impact journal, and asked how this impacted the model’s prediction on future citations. As expected, the model learned that 99th percentile journals will on average yield an above-average number of citations, while a 1st percentile journal yields below-average citations. Interestingly, a median journal impact factor was found to be sufficient in producing articles with just as many citations as 99th percentile journals.
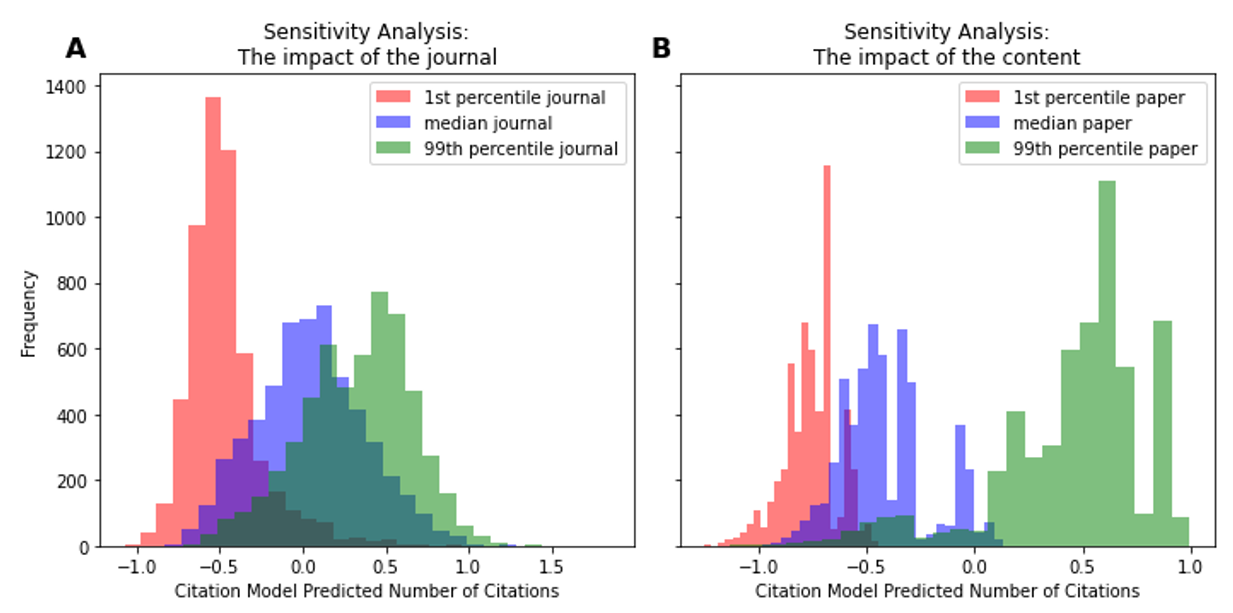
Exhibit 3: Sensitivity analyses comparing how the OE High Impact predicted number of citations varies depending on (A) the strength of the journal, and (B) the strength of the content.
In Exhibit 3B, we performed the reverse perturbation and artificially mutated every publication to have the same content as a highly cited paper, a median paper, and a poorly cited paper, and asked the model how content (as compared to where it was published) influenced an article’s predicted number of citations. Here we can see that the model appears to be much more sensitive to article content. A 99th percentile article is almost always predicted to produce an above-average level of citations, irrespective of journal metrics. By contrast, a median, or low-citation article tends to produce fewer than average citations. Collectively, these sensitivity analyses suggest that the OE impact model believes that what an article says, and not where it was published, defines the impact an article will have.
Finally, leveraging the treatment tagging system of OE M.I.N.D. we asked which treatment tags were associated with the highest predicted 5-year citation count. The results are summarized in Exhibit 4. Among this list are colloquial “hot” topics in orthopedics, including stem cell research, cannabis, platelet rich plasma, and decompressive laminectomy, and others.
Exhibit 4: Treatments associated with the highest number of predicted citations per the citation prediction model.
Rank | Treatment | Average # of Predicted Citations at 5 years Post Publishing |
1 | Autologous Chondrocyte Implantation | 43.8 |
2 | Tetrahydrocannabinol (THC) | 35.0 |
3 | Cannabidiol (CBD) | 31.5 |
4 | Microfracture | 31.5 |
5 | Tanezumab | 31.2 |
6 | Mesynchymal Stem Cells | 30.5 |
7 | Chemotherapy | 27.8 |
8 | Platelet-Rich Plasma (PRP) | 27.4 |
9 | Decompressive Laminectomy | 26.9 |
10 | Anticoagulants | 26.1 |
Added value for the reader: the OE High Impact Tag
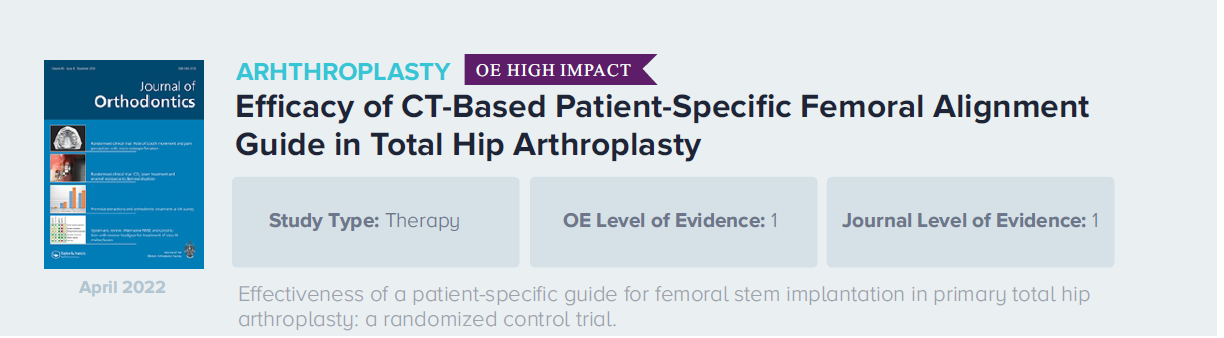
Exhibit 5: The OE High Impact Tag will be available soon on ACE Reports to identify significantly impactful works.
We therefore present OE High Impact (Exhibit 5). As we launch this new feature, some ACE reports will begin to display “OE High Impact” above their title. This tag indicates our belief that the identified paper provides important, impactful data, and thus, will ultimately prove to be highly cited. It’s important to note that no measure of impact is perfect. Thus, articles that are not labelled as “high-impact” should not be construed as being of poor quality and undeserving of attention. To reiterate the words of Nobel Laureate Sydney Brenner, “What matters absolutely is the scientific content of a paper, and nothing will substitute for either knowing or reading it”. Nevertheless, in revisiting the initial problem, how can clinicians better stay up to date on research? OE High Impact offers a searchable indicator that can help readers to cut through the noise, and refine their focus to potentially innovative works.
Contributors
References
- Haynes RB, McKibbon KA, Fitzgerald D, Guyatt GH, Walker CJ, Sackett DL. How to keep up with the medical literature: II. Deciding which journals to read regularly. Ann Intern Med [Internet]. 1986;105(2):309–12. Available from: https://annals.org
- Seglen PO. Why the impact factor of journals should not be used for evaluating research. BMJ [Internet]. 1997 Feb 15;314(7079):497. Available from: https://www.bmj.com/content/314/7079/497.1
- Holzer LA, Holzer G. The 50 Highest Cited Papers in Hip and Knee Arthroplasty. J Arthroplasty. 2014 Mar 1;29(3):453–7.
© OrthoEvidence Inc. All Rights Reserved.